Longitudinal study design poses a number of challenges; they are costly, time-consuming, and need extensive resources. However, findings derived from longitudinal studies are generally regarded as more robust than case-control and cross-sectional designs, especially with regards to determining causality. A common issue in every prospective cohort study is loss to follow-up, or also known as attrition. This refers to the situation where responders at baseline drop out, or stop participating in the study during the follow-up period. The phenomenon of loss to follow-up can be due to various reasons: death, migration (or relocation), refusal to continue, loss of interest, and many more. Some studies suggested 20% as the cut-off for minimum attrition rate in a cohort study for the results to be valid. But in reality, loss to follow-up is often higher than that.
A large number of drop outs causes several problems. First, it can reduce the power of a study if the number of responders at follow-up is way below the initial supposed (calculated) sample size. Second, it can lead to potential bias in the results if non-responders are characteristically different than responders. For instance, if those who drop out during the follow-up period comprise a certain ethnic group or come from a particular locality, the sample might no longer be representative as it initially was. Or if those lost to follow-up comprise the less educated, poorer and sicker group, the remaining respondents are in fact, the healthier and wealthier ones, and may not represent the true characteristics of the sampling frame. This gives rise to what is called the ‘healthy survivor effect’.
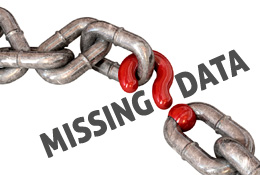
The best way to avoid high attrition is prevention, which requires thorough planning prior to embarking on the study. The researcher should plan all possible efforts or methods that can be undertaken to minimize drop-outs as much as possible. In order to do that, he/ she must be able to foresee potential obstacles that can lead to drop-outs. However, when loss to follow-up has occurred, the next step would be to exercise caution and adopt specific approaches to data analysis. The initial step is to compare the basic characteristics between responders and non-responders, and determine whether or not they are significantly different. Then, one needs to quantify the missing data, and try to identify its type or mechanism (usually with the help of statistical software).
The three common types or mechanism of missing data are: 1) Missing Completely at Random (MCAR); 2) Missing at Random (MAR), and; 3) Missing not at Random (MNAR). If the pattern of missingness is found to be MCAR, most studies agree that the possibility of bias in results is low. Therefore, the researcher can proceed with conventional analysis, as planned. If the missingness is MAR, a number of steps can be taken such as performing data imputation and sensitivity analysis. Imputation of data is a process where missing values are imputed, or given several potential estimates based on observed data distribution so that analysis can then be run with the assumption that information is complete. Sensitivity analysis in this context refers to making a minimum of two sets of analysis – one with imputed data and one excluding missing data – and compare between the two. If the results are not so different, we can conclude that attrition is unlikely to cause substantial bias to results. On the other hand, if there are large discrepancies, or if the missingness is found to be MNAR, conventional analysis may not suffice; one may have to resort to other more advanced statistical methods which are beyond the scope of this article.
Sharing by,
Research and Publications Unit,
IKRAM Health